
VOINEA Camelia Florela
- Department of Public Policy, International Relations and Security Studies, Faculty of Political Science, University of Bucharest, Bucharest, Romania
- Adaptive networks, Algorithms for Network Analysis, Collaboration in networks, Community structure in networks, Complex networks mining, Dynamics on networks, Emergence in complex networks, Internet and World Wide Web, Knowledge and innovation networks, Multi-agent based model, Multilayer, multiplex or multilevel Networks, Networks in neuroscience, Nonlinear dynamics in networks, Online social networks, Philosophy of network complexity, Political networks, Resilience and robustness in networks, Self-organization in complex networks, Semantic Social Networks, Social influence analysis, Social networks, Spreading
- recommender
Recommendations: 0
Review: 1
Review: 1
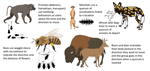
Improving human collective decision-making through animal and artificial intelligence
Bio-inspired solutions for collective decision-making in a networked society
Recommended by Frédéric Amblard based on reviews by Camelia Florela Voinea and 1 anonymous reviewerFormal voting biases and weaknesses like Arrow's or Condorcet's paradoxes are known for quite a long time, but they are often considered as curiosities for mathematicians and cases that rarely occur in practice. Although, recent elections underlined the actual weaknesses of our collective decision-making processes, either concerning the resulting lack of representativity (people getting elected but representing actually a minority of the population) or more globally the efficiency of the decisions taken. A significant gap exists in between on one hand the opinions of a networked population that are structured and evolve using social media, leading to a much more visible diversity and on the other hand the ones of representatives that are still structured by political parties. Such a situation leads to questioning the processes used to choose representatives and more globally to make collective decision-making with alternatives that are proposed more and more (liquid democracy for instance (Blum and Zuber, 2016).
The article by Sueur et al. shed new light on the situation by proposing to examine further the solutions selected along time by the animal kingdom in order to make collective decision-making. Although they advocate that the decisions taken are not the same (decision for an animal group to move to another place) and do not involve the same cognitive abilities at the individual level, the existing processes could get adapted to human contexts. One of the most striking advantages of such bio-inspired approach is that animal collective decision-making processes are robust and enable to manage conflicting views, diversity of opinions and avoid forms of despotism that are not much present in animals, rather consensual decision-making being the norm. Another argument, probably less put forward by the authors is that such solutions may scale well with large networked populations as they exist for some animal species. Therefore it looks like we could have a kind of readymade library of processes for collective decision-making that are yet efficient to make timely decisions for different purposes with different population sizes and structures.
Their argument is consolidated by the possibility of using AI technologies in order to enable to support the adaptation of such solutions. Without building explicitly the link, they identify that AI could be used in order to guarantee a fair process and to scale up the proposed solutions at the level of massive populations.
This is probably the less convincing part of the proposal and it concerns the relation between human and AI. Even if the authors admit that the acceptance of AI solutions by part of the population is a key issue, as it concerns directly the legitimacy of the process and the compliance of the population with the resulting decision. They tend to minimize the gap to fill before having a fair AI with potential behavior that can be verified before using it with confidence to support a democratic process of decision-making.
Nevertheless, the article brings forward good arguments, well formalized using relevant concepts for the use of bio-inspired solutions for collective decision-making.
References
Blum C, Zuber CI (2016) Liquid Democracy: Potentials, Problems, and Perspectives. Journal of Political Philosophy, 24, 162–182. https://doi.org/10.1111/jopp.12065
Sueur C, Bousquet C, Espinosa R, Deneubourg J-L (2021) Improving human collective decision-making through animal and artificial intelligence. hal-03299087, ver. 3 recommended and peer-reviewed by Peer Community in Network Science. https://hal.archives-ouvertes.fr/hal-03299087