Latest recommendations
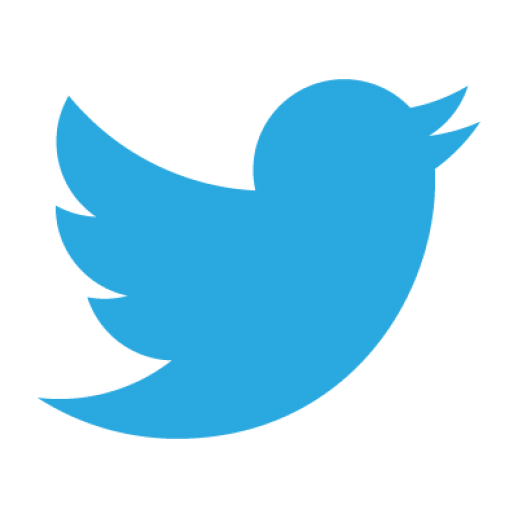
Id | Title * ▼ | Authors * | Abstract * | Picture * | Thematic fields * | Recommender | Reviewers | Submission date | |
---|---|---|---|---|---|---|---|---|---|
27 Jan 2024
![]() A single changing hypernetwork to represent (social-)ecological dynamicsCedric Gaucherel, Maximilien Cosme, Camille Nous, Franck Pommereau https://doi.org/10.1101/2023.10.30.564699The Dawn of Dynamic Hypergraph Modelling in EcologyRecommended by Cédric SueurThe study of Gaucherel et al. (2024) represents a groundbreaking shift in the field of ecosystem representation and management (DeFries and Nagendra 2017), offering a comprehensive and innovative approach that emphasises the importance of dynamic and complex models to accurately understand and preserve our ecosystems. At the heart of this pioneering work is the introduction of advanced representational methods such as interaction networks and hypergraphs (Bretto 2013; Golubski et al. 2016), which mark a significant departure from traditional static models. These novel representations are adept at capturing the intricate, multi-component interactions within ecosystems, thereby providing a much more nuanced and interconnected view of ecological systems. This approach is particularly innovative as it moves beyond the simplicity of previous models, offering a dynamic, fluid, and interconnected perspective of ecological dynamics that is more reflective of the real-world complexity of these systems. Furthermore, the study proposes the integration of social networks with ecological ones (Sosa et al. 2021; Sueur 2023), acknowledging the profound impact that human activities have on natural systems (Afana 2021; Elmqvist et al. 2021; Pelé et al. 2021). This interdisciplinary approach is pioneering in its attempt to bridge the gap between social and ecological studies, underscoring the interconnectedness of natural and human systems and highlighting the need for a holistic approach to ecosystem management (Stokols et al. 2013; Stone-Jovicich 2015). The significance of the study lies not only in its methodological innovations but also in the implications it holds for the field of ecology and environmental management. By employing these advanced methodologies, the study provides a more thorough understanding of ecosystems. By considering a wide range of components and their interactions, these models offer insights into the complex dynamics of ecosystems, which are crucial for developing effective conservation and management strategies. This comprehensive approach is particularly important in an era where ecosystems are increasingly threatened by a variety of factors, including climate change, habitat destruction, and pollution (Mantyka‐pringle et al. 2012; Trathan et al. 2015). Additionally, the dynamic nature of the proposed models, especially the use of hypergraphs, facilitates the adaptive management of ecosystems. By accurately representing the changing interactions and components within these systems, these models enable managers and policymakers to respond more effectively to ecological changes, ensuring that conservation efforts are both effective and timely (Ascough Ii et al. 2008; Fischer et al. 2009; McKinley et al. 2017). Moreover, the advanced modelling techniques proposed by the study have the potential to significantly improve predictive capabilities regarding ecosystem dynamics. Understanding the complex interactions and the long-term dynamics of ecosystems allows for better anticipation of future changes and challenges, a crucial aspect in a rapidly changing world where ecosystems are under constant threat. In conclusion, this study marks a significant advancement in the field of ecological representation and management. Its innovative approach in utilising complex models like hypergraphs and integrating social and ecological networks provides a more comprehensive, dynamic, and nuanced understanding of ecosystems. Such innovations are crucial in an era of rapid environmental change and increasing anthropogenic pressures. By enhancing our ability to understand, predict, and manage ecosystem dynamics, this study lays the groundwork for more effective conservation strategies and ecosystem management practices. It underscores the need for a holistic approach to understanding and preserving our natural world, recognising the intricate and interconnected nature of ecosystems and the pivotal role humans play within them. References Afana R (2021) Ecocide, Speciesism, Vulnerability: Revisiting Positive Peace in the Anthropocene. In: Standish K, Devere H, Suazo A, Rafferty R (eds) The Palgrave Handbook of Positive Peace. Springer, Singapore, pp 1-18 Ascough Ii J, Maier H, Ravalico J, Strudley M (2008) Future research challenges for incorporation of uncertainty in environmental and ecological decision-making. Ecol Model 219:383-399 Bretto A (2013) Hypergraph theory. Introd Math Eng Cham Springer 1: DeFries R, Nagendra H (2017) Ecosystem management as a wicked problem. Science 356:265-270 Elmqvist T, Andersson E, McPhearson T, et al (2021) Urbanization in and for the Anthropocene. Npj Urban Sustain 1:6 Fischer J, Peterson GD, Gardner TA, et al (2009) Integrating resilience thinking and optimisation for conservation. Trends Ecol Evol 24:549-554 Gaucherel C, Cosme M, Noûs C, Pommereau F (2024) A single changing hypernetwork to represent (social-)ecological dynamics. bioRxiv, 2023.10.30.564699, ver. 3 peer-reviewed and recommended by Peer Community in Network Science. Golubski AJ, Westlund EE, Vandermeer J, Pascual M (2016) Ecological networks over the edge: hypergraph trait-mediated indirect interaction (TMII) structure. Trends Ecol Evol 31:344-354 Mantyka‐pringle CS, Martin TG, Rhodes JR (2012) Interactions between climate and habitat loss effects on biodiversity: a systematic review and meta‐analysis. Glob Change Biol 18:1239-1252 McKinley DC, Miller-Rushing AJ, Ballard HL, et al (2017) Citizen science can improve conservation science, natural resource management, and environmental protection. Biol Conserv 208:15-28 Pelé M, Georges J-Y, Matsuzawa T, Sueur C (2021) Editorial: Perceptions of Human-Animal Relationships and Their Impacts on Animal Ethics, Law and Research. Front Psychol 11: Sosa S, Jacoby D, Lihoreau M, Sueur C (2021) Animal social networks: Towards an integrative framework embedding social interactions, space and time. Methods Ecol Evol 12:4-9 Stokols D, Lejano RP, Hipp J (2013) Enhancing the Resilience of Human-Environment Systems: a Social Ecological Perspective. Ecol Soc 18: Stone-Jovicich S (2015) Probing the interfaces between the social sciences and social-ecological resilience: insights from integrative and hybrid perspectives in the social sciences. Ecol Soc 20: Sueur C (2023) Socioconnectomics: Connectomics Should Be Extended to Societies to Better Understand Evolutionary Processes. Sci 5:5. https://doi.org/10.3390/sci5010005 Trathan PN, García‐Borboroglu P, Boersma D, et al (2015) Pollution, habitat loss, fishing, and climate change as critical threats to penguins. Conserv Biol 29:31-41 | A single changing hypernetwork to represent (social-)ecological dynamics | Cedric Gaucherel, Maximilien Cosme, Camille Nous, Franck Pommereau | <p style="text-align: justify;">To understand and manage (social-)ecological systems, we need an intuitive and rigorous way to represent them. Recent ecological studies propose to represent interaction networks into modular graphs, multiplexes and... | ![]() | Algorithms for Network Analysis, Biological Networks, Community structure in networks, Ecological networks, Geometry and topology of networks or graphs, Network models, Qualitative methods in network analysis, Social networks, Temporal networks | Cédric Sueur | 2023-11-03 17:18:11 | View |
MANAGING BOARD
Eleni Akrida
Corinne Bonnet
Alecia Carter
Michel Grossetti
Norbert Hounkonnou
Matthieu Latapy
Brenda McCowan
Elisa Omodei
Cédric Sueur